《强化学习——Intrinsic Curiosity Module》
作者:互联网
强化学习——Intrinsic Curiosity Module
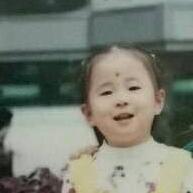
其他强化学习资料
【1】搬砖的旺财:David Silver 增强学习——笔记合集(持续更新)
目录
1、拓展
2、论文
3、前言
4、Reward Shaping
····4.1 小孩的例子
····4.2 训练计算机玩VizDoom游戏的例子
····4.3 训练机械臂的例子
5、好奇心架构
6、损失函数
7、代码解读
····7.1 network
····7.2 ICM
····7.3 Loss
8、参考文献
1、拓展
李宏毅老师的DRL课程,受益匪浅:
PPT:http://speech.ee.ntu.edu.tw/~tlkagk/courses/MLDS_2018/Lecture/PPO%20(v3).pdf
视频:https://www.bilibili.com/video/av24724071/?p=7
2、论文
- Large-Scale Study of Curiosity-Driven Learning
- Curiosity-driven Exploration by Self-supervised Prediction
- Curiosity-driven Exploration for Mapless Navigation with Deep Reinforcement Learning
第三篇论文我在写毕设的绪论时,曾经调研过,来看当时怎么写的:
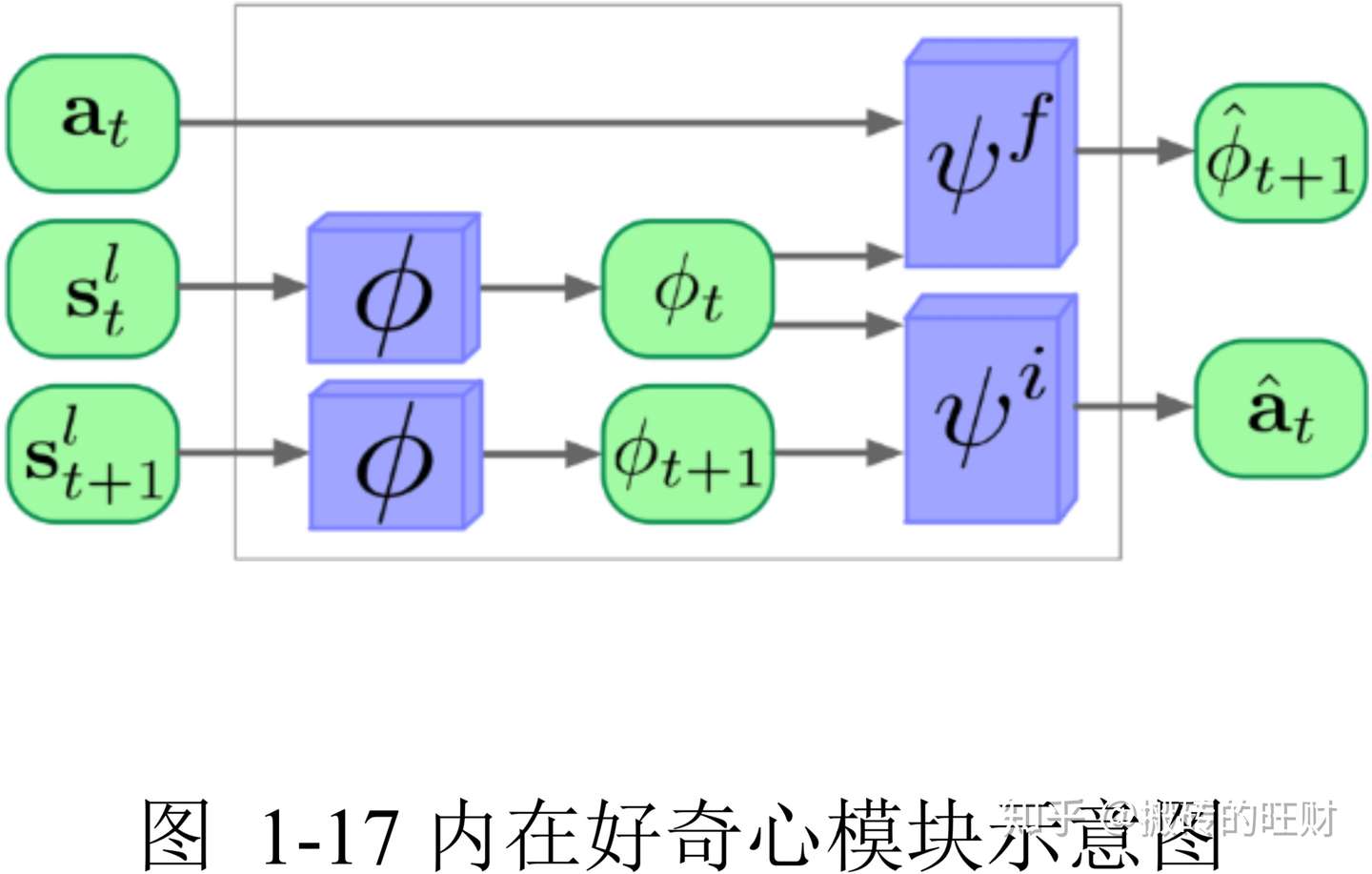


3、前言
为了解决RL中reward过于稀疏的问题,引入三种解决方法:
- Reward Shaping
- Curriculum Learning
- Hierarchical Reinforcement Learning
内在好奇心模块(Intrinsic Curiosity Module, ICM)属于第一种,来看看它的神奇功效~
4、Reward Shaping
- 4.1 小孩的例子
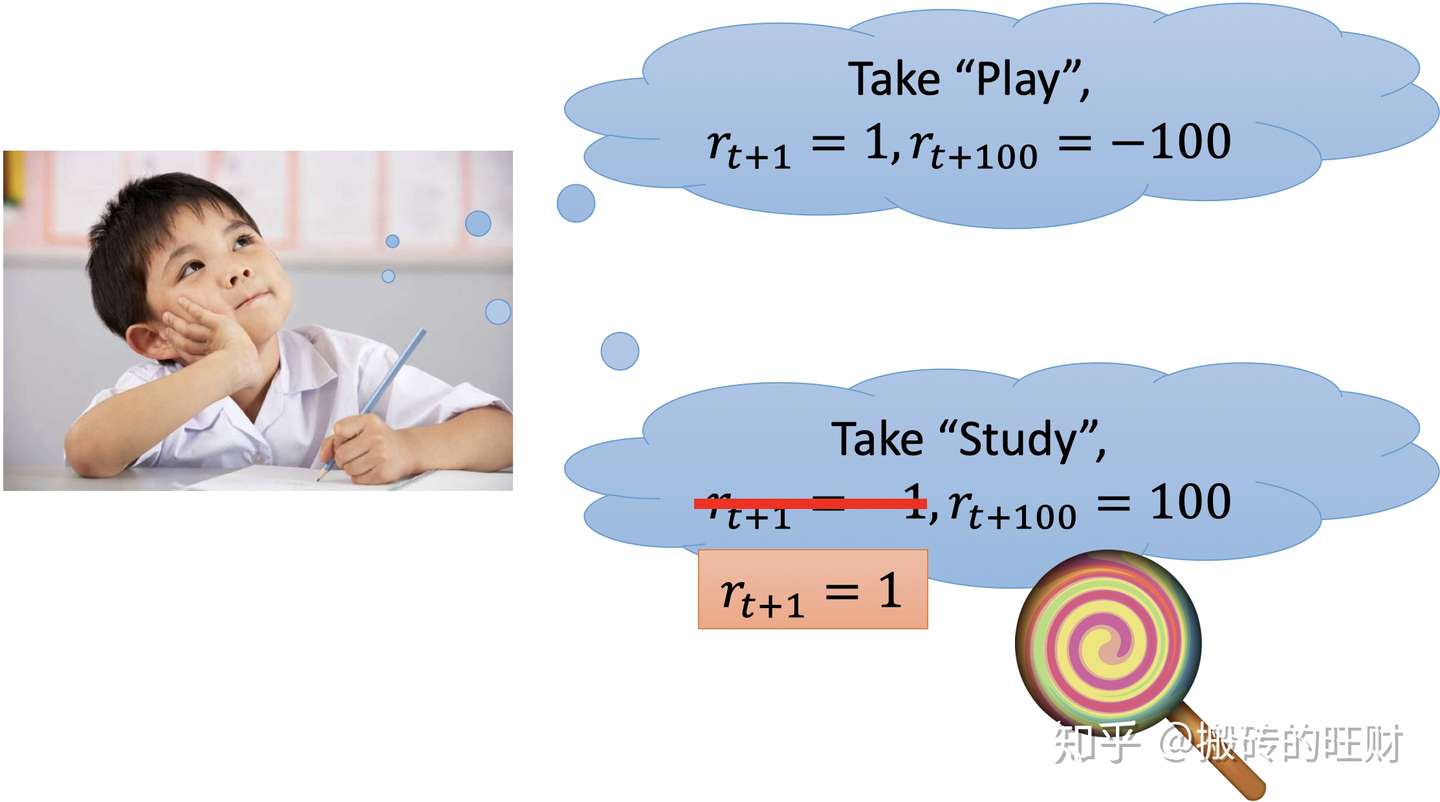
小孩如果想要“Play”,他获取了快乐,精神比较愉悦,于是及时reward为+1,但是因为“贪玩”导致考试不及格,于是往后一百步的rewrd为-100;
小孩如果想要“Study”,他觉得不是很开心,于是及时reward为-1,但是因为勤奋学习考试取得了好成绩,于是往后一百步的rewrd为100。
为了鼓励小孩去“Study”,这时候可以重新塑造这个学习的reward为+1。
- 4.2 训练计算机玩VizDoom游戏的例子
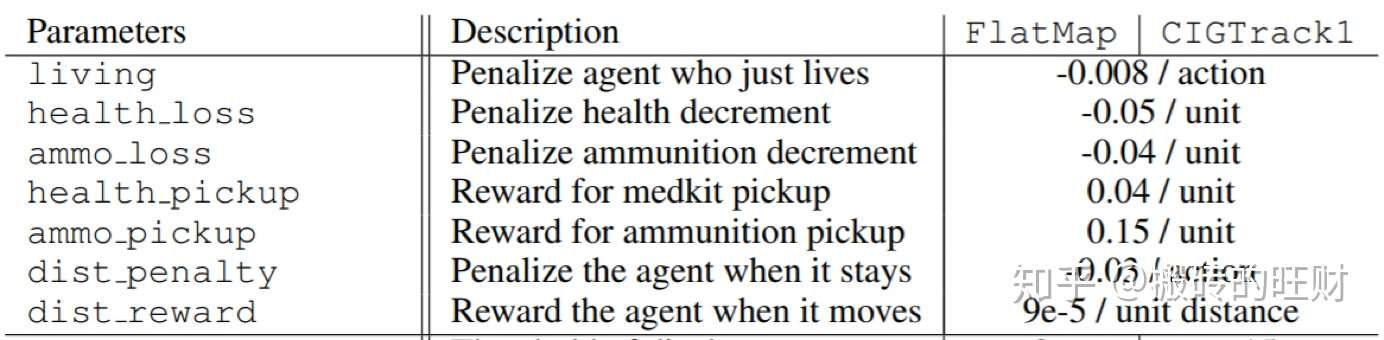
这个游戏本身只有一个最终的reward,赢就是正奖励,输就是负奖励。因为过于稀疏,所以人为的去设置一些Reward Shapping,比如加到血袋会有一个小奖励,加到一个枪会有一个小奖励,打枪不中会有一个负的小奖励,从而使得reward不那么稀疏。
(注:这些奖励的值是调参试出来的~)
- 4.3 训练机械臂的例子
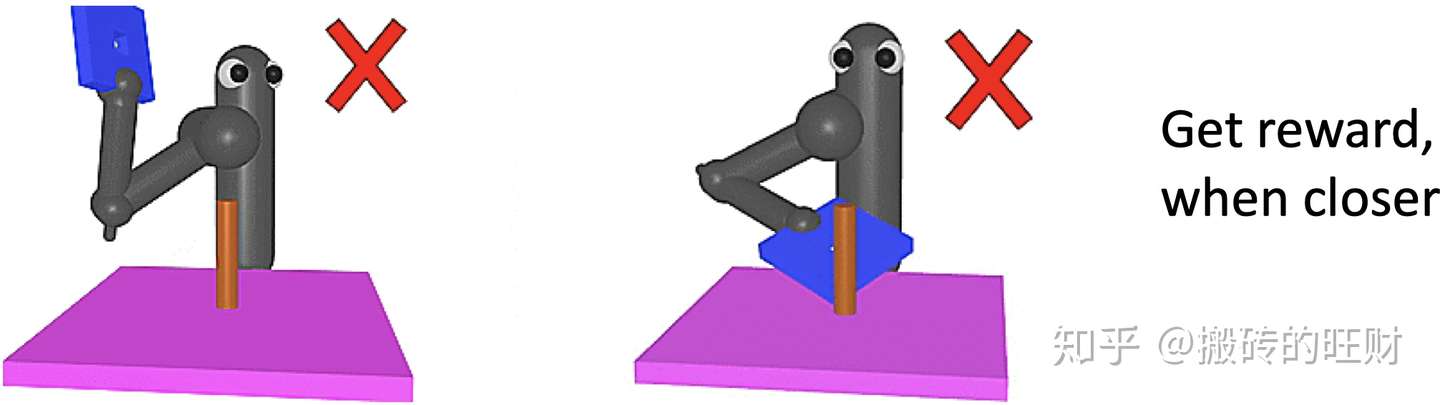
Reward Shapping有时也会出错,比如说训练机械臂拿着蓝色的板穿过红色的柱子。Reward Shapping是:蓝色小框架越接近木头架,重塑奖励越大,但是有时候机器会一直击打着这个架子。
5、好奇心架构
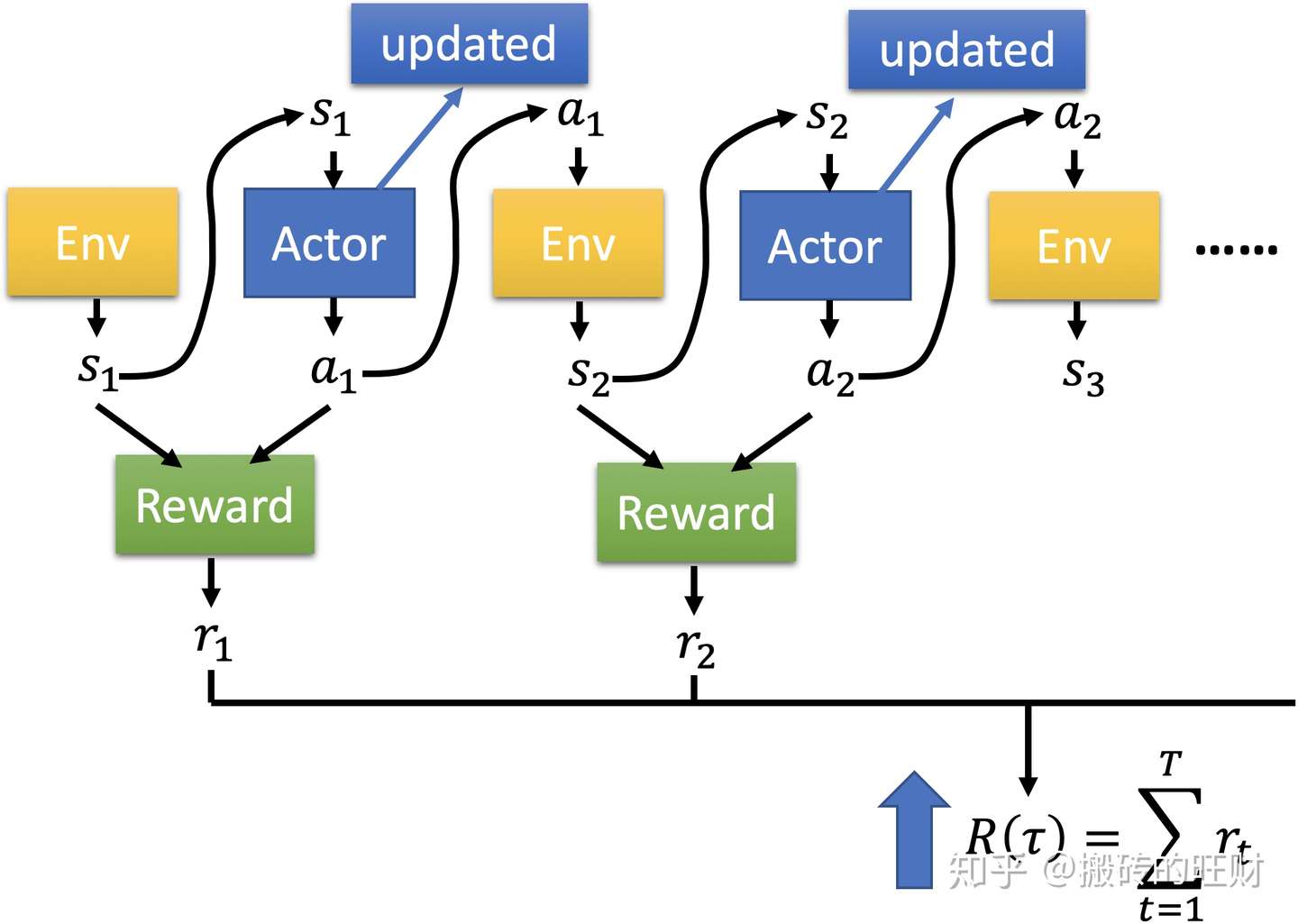
首先,回顾一下之前Actor与Environment交互的过程:
Env产生一个state输入Actor,Actor再产生action
作用于Env,由此得到一个Reward
,循环往复,就可以得到轨迹
,以及它对应的
。
也就是说,一个 ,一个
去得到一个奖励
。
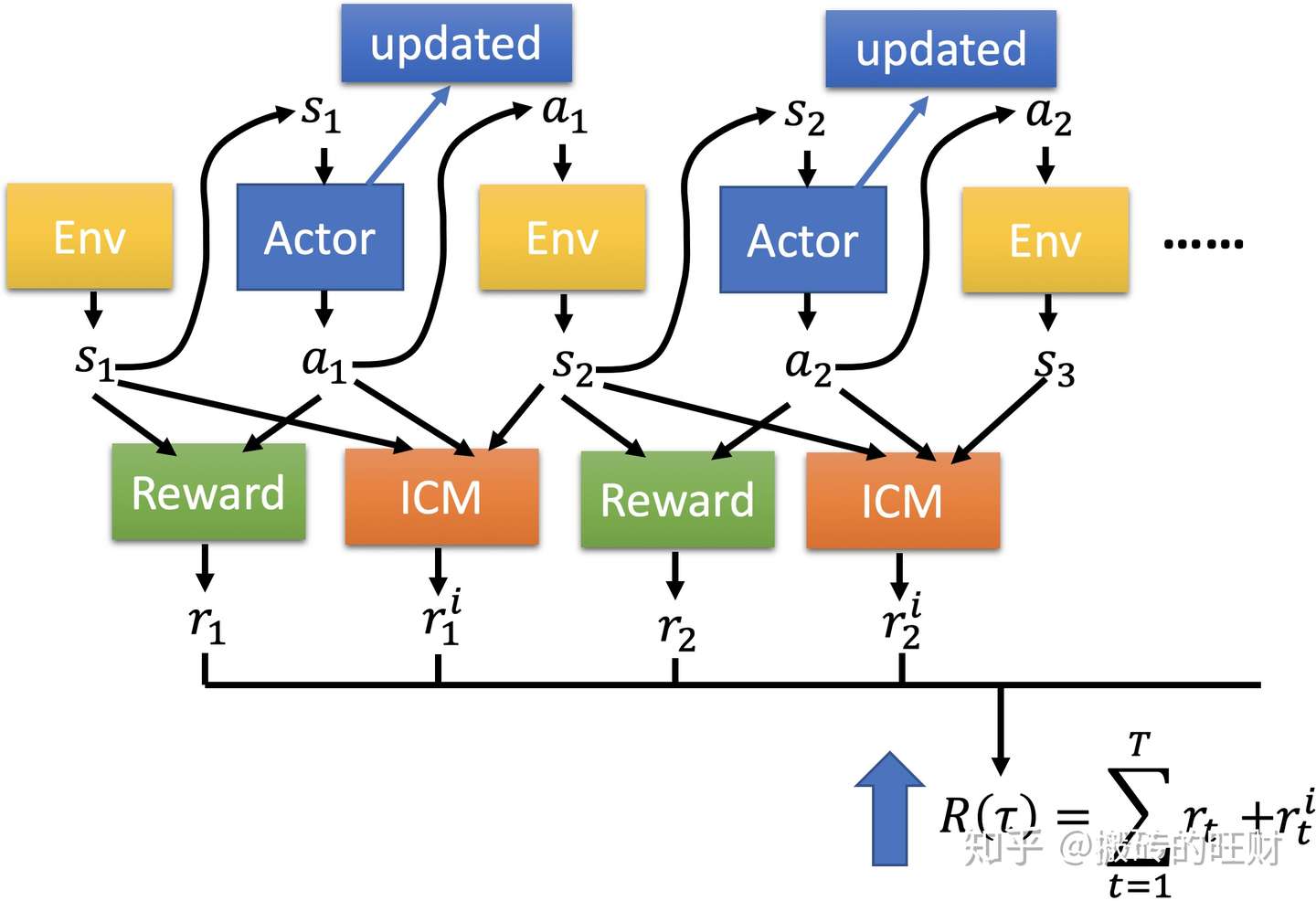
但是,增加ICM模块后,随之增加了一个好奇心的奖励。也就是说,一个 ,一个
进入网络后得到两个奖励,分别是
和
,
是Env给的,
是ICM给的,RL的目的是使两个奖励的加和达到最大。
接下来,分析一下ICM内部是如何工作的,模型如下图:
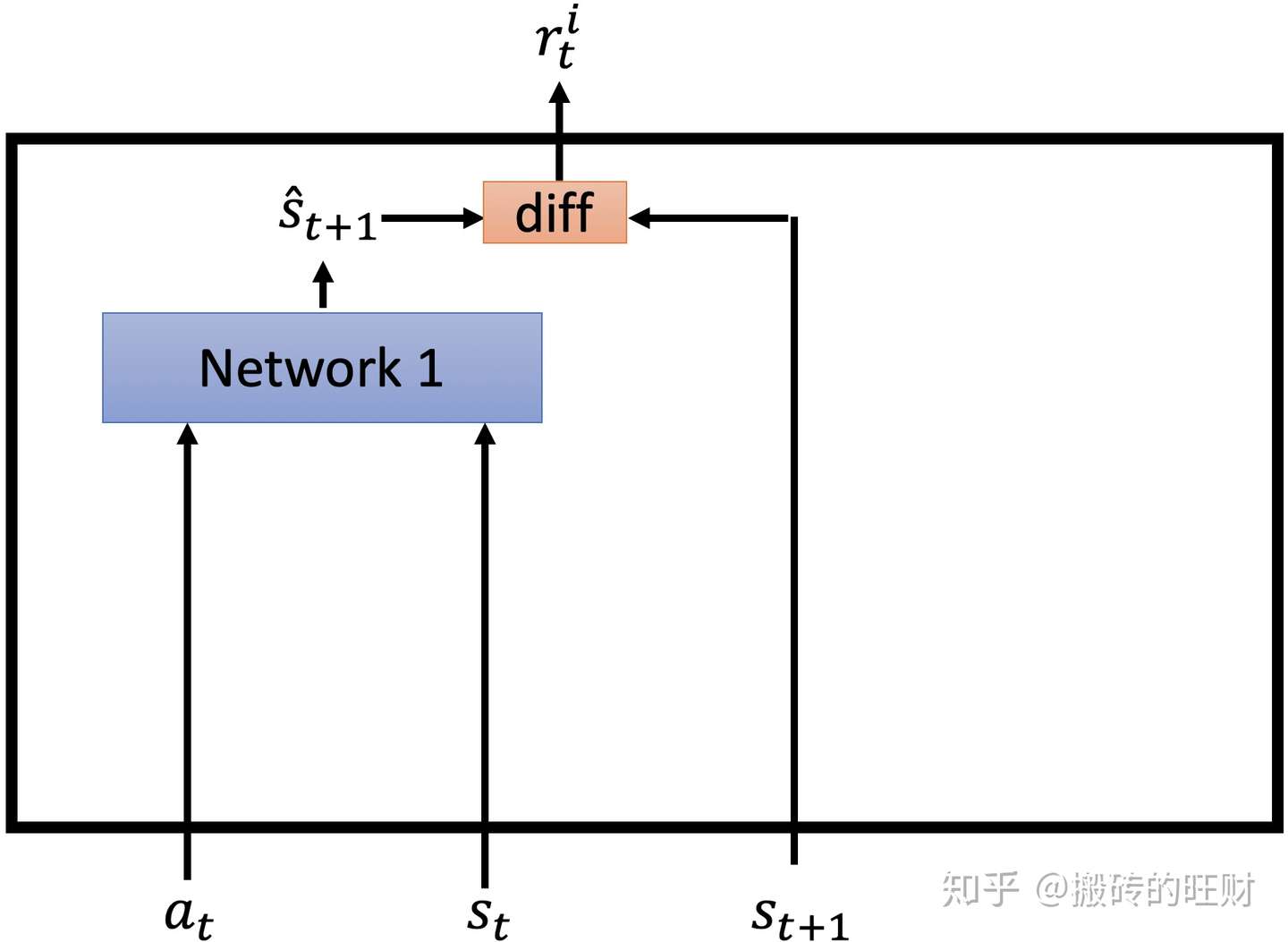
输入有三个, ,
,
。
,
输入Network1得到一个预估的
,判断
和
的区别大小,如果区别大的话,
就很大,如果区别小的话,
就很小。
该模型鼓励冒险,希望Agent会做一些难以预料的事情。
但是这种情况也是有弊端的。
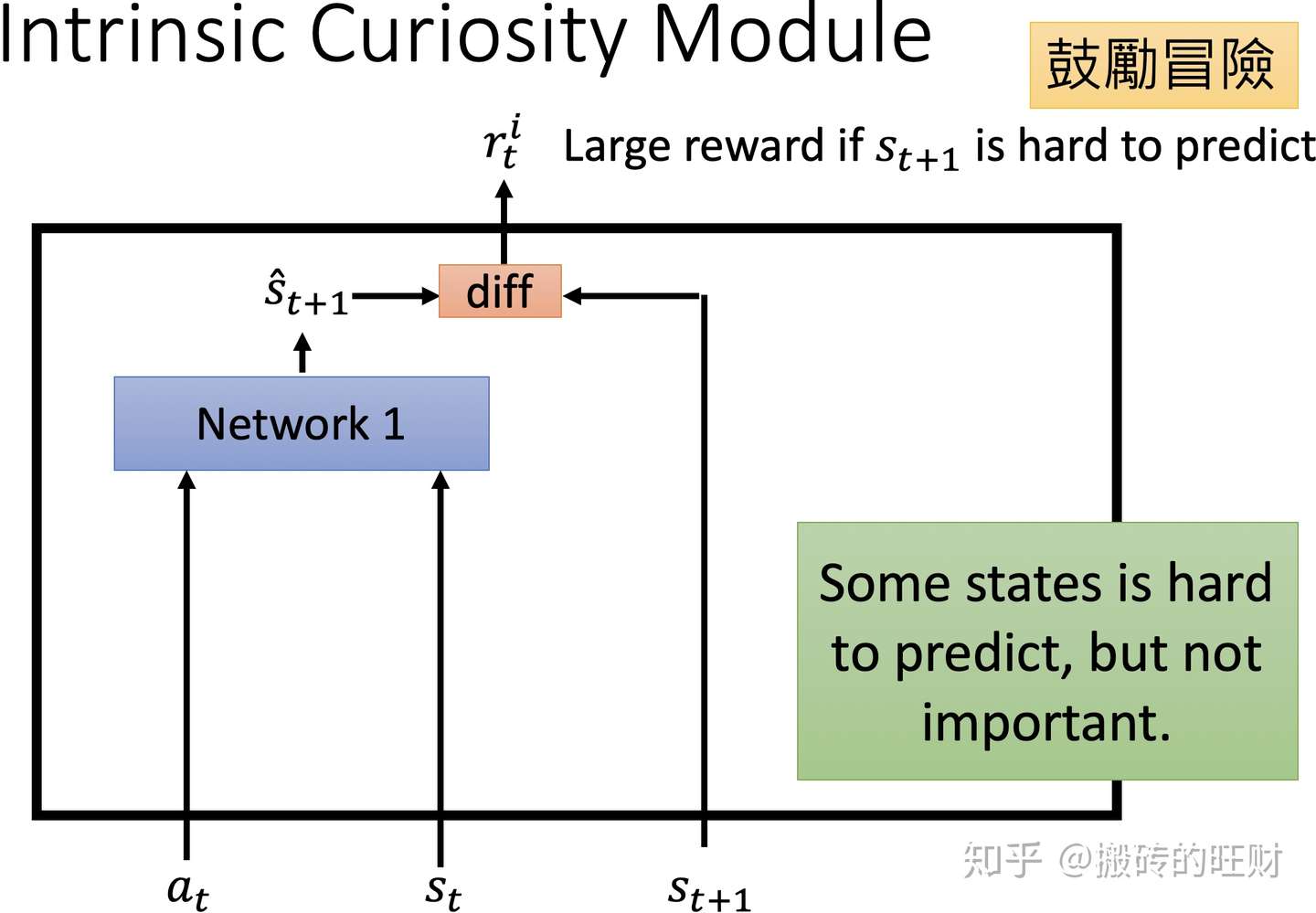
比如Agent在玩游戏,但是游戏画面的背景是树叶在舞动,树叶如何舞动是一个难以预料的东西,这个模型会鼓励这种难以预料的东西。所以这个模型会让Agent一直观察树叶是如何舞动。
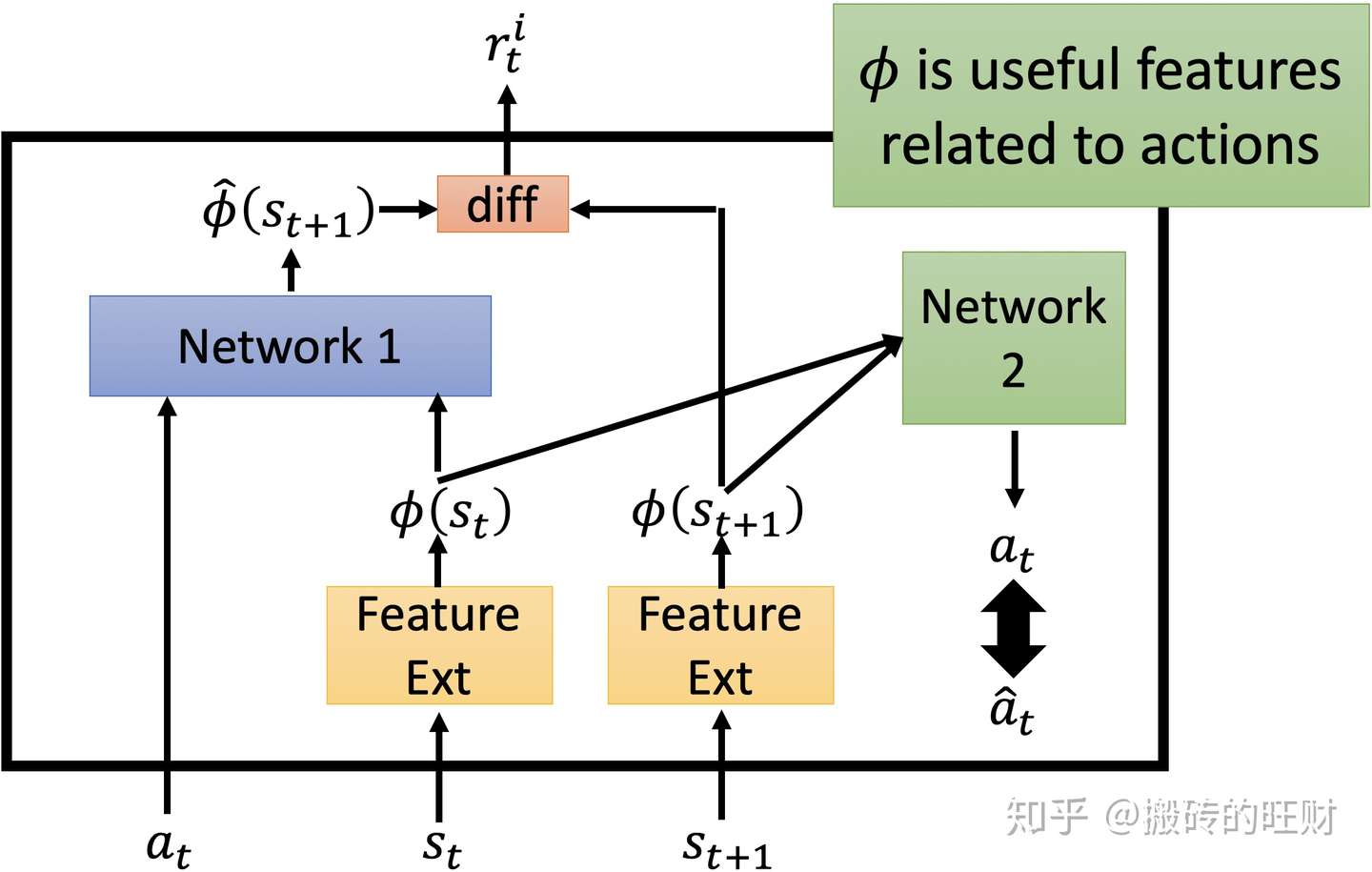
所以,不能让Agent总看一些无关紧要的东西,于是,增加Network2,用来判断这个东西是否与实验结果相关。
输入是 ,
,
。
和
会经过Feature Ext,从而生成两个向量
和
,将这两个向量输入到Network2中,生成对
的预测
,比较
和
的关系,如果区别不大,证明这个东西是和结果有关系的,但是如果区别大,就证明这是一个无关紧要的东西。Network1和之前保持一致。
6、损失函数
设计一个神经网络(Neural Network, NN)对当前状态 做特征抽取
,对下一状态
做特征抽取
,来预测在这两个状态间采用的action:
。
通过最小化 与实际动作
的误差,利用反向传播让NN学习到真正由action影响的特征。
因为这里的action是离散的,所以可以对 做soft-max,然后通过maximum likelihood estimation来设置对应的loss函数,即
。
在提取出特征 后,同样可以使用NN来预测下一个状态
的特征
:
。
因为预测出来的特征是个向量,而且也不清楚向量中的每个元素代表什么,所以一个很直观的想法就是利用 范数来作为loss:
。
同时,我们可以利用上面的损失 来构造curiosity reward:
。
最后,Agent的学习目标为: 。
其中 ,
,作为相应项的尺度的衡量。
那么在训练的时候,因为不断对 做优化,所以最开始部分预测准确之后,为了获得更多的curiosity reward就会主动地去探索更多的未知的状态。
7、代码解读
运用ICM解决Atari游戏,完整代码请点击:还没有debug完成,稍后上传~
- 7.1 network
def network(self, network_name):
# Input
x_image = tf.placeholder(tf.float32, shape = [None,
self.img_size,
self.img_size,
self.Num_stacking * self.Num_colorChannel])
x_normalize = (x_image - (255.0/2)) / (255.0/2)
with tf.variable_scope(network_name):
# Convolution variables
w_conv1 = self.conv_weight_variable('_w_conv1', self.first_conv)
b_conv1 = self.bias_variable('_b_conv1',[self.first_conv[3]])
w_conv2 = self.conv_weight_variable('_w_conv2',self.second_conv)
b_conv2 = self.bias_variable('_b_conv2',[self.second_conv[3]])
w_conv3 = self.conv_weight_variable('_w_conv3',self.third_conv)
b_conv3 = self.bias_variable('_b_conv3',[self.third_conv[3]])
# Densely connect layer variables
w_fc1 = self.weight_variable('_w_fc1',self.first_dense)
b_fc1 = self.bias_variable('_b_fc1',[self.first_dense[1]])
w_fc2 = self.weight_variable('_w_fc2',self.second_dense)
b_fc2 = self.bias_variable('_b_fc2',[self.second_dense[1]])
# Network
h_conv1 = tf.nn.relu(self.conv2d(x_normalize, w_conv1, 4) + b_conv1)
h_conv2 = tf.nn.relu(self.conv2d(h_conv1, w_conv2, 2) + b_conv2)
h_conv3 = tf.nn.relu(self.conv2d(h_conv2, w_conv3, 1) + b_conv3)
h_flat = tf.reshape(h_conv3, [-1, self.first_dense[0]])
h_fc1 = tf.nn.relu(tf.matmul(h_flat, w_fc1)+b_fc1)
output = tf.matmul(h_fc1, w_fc2) + b_fc2
return x_image, output
- 7.2 ICM
# Intrinsic Curiosity Module
def ICM(self):
# Input
s_current = tf.placeholder(tf.float32, shape = [None,
self.img_size,
self.img_size,
self.Num_stacking * self.Num_colorChannel])
s_current = (s_current - (255.0/2)) / (255.0/2)
s_next = tf.placeholder(tf.float32, shape = [None,
self.img_size,
self.img_size,
self.Num_stacking * self.Num_colorChannel])
s_next = (s_next - (255.0/2)) / (255.0/2)
with tf.variable_scope('curiosity'):
# Convolution variables
w_conv1 = self.conv_weight_variable('_w_conv1', [3,3,self.Num_stacking * self.Num_colorChannel,32])
b_conv1 = self.bias_variable('_b_conv1',[32])
w_conv2 = self.conv_weight_variable('_w_conv2', [3,3,32,32])
b_conv2 = self.bias_variable('_b_conv2',[32])
w_conv3 = self.conv_weight_variable('_w_conv3', [3,3,32,32])
b_conv3 = self.bias_variable('_b_conv3',[32])
w_conv4 = self.conv_weight_variable('_w_conv4', [3,3,32,32])
b_conv4 = self.bias_variable('_b_conv4',[32])
# Feature Vector
s_conv1 = tf.nn.elu(self.conv2d(s_current, w_conv1, 2) + b_conv1)
s_conv2 = tf.nn.elu(self.conv2d(s_conv1, w_conv2, 2) + b_conv2)
s_conv3 = tf.nn.elu(self.conv2d(s_conv2, w_conv3, 2) + b_conv3)
s_conv4 = tf.nn.elu(self.conv2d(s_conv3, w_conv4, 2) + b_conv4)
s_conv4_flat_dim = s_conv4.shape[1]*s_conv4.shape[2]*s_conv4.shape[3]
s_conv4_flat = tf.reshape(s_conv4, [tf.shape(s_conv4)[0], s_conv4_flat_dim])
s_next_conv1 = tf.nn.elu(self.conv2d(s_next, w_conv1, 2) + b_conv1)
s_next_conv2 = tf.nn.elu(self.conv2d(s_next_conv1, w_conv2, 2) + b_conv2)
s_next_conv3 = tf.nn.elu(self.conv2d(s_next_conv2, w_conv3, 2) + b_conv3)
s_next_conv4 = tf.nn.elu(self.conv2d(s_next_conv3, w_conv4, 2) + b_conv4)
s_next_conv4_flat_dim = s_next_conv4.shape[1]*s_next_conv4.shape[2]*s_next_conv4.shape[3]
s_next_conv4_flat = tf.reshape(s_next_conv4, [tf.shape(s_next_conv4)[0], s_next_conv4_flat_dim])
# Forward Model
a_t = tf.placeholder(tf.float32, shape = [None, self.Num_action])
input_forward = tf.concat([s_conv4_flat, a_t], 1)
forward_fc1 = tf.layers.dense(input_forward, 256, activation=tf.nn.relu)
forward_fc1 = tf.concat([forward_fc1, a_t], 1)
forward_fc2 = tf.layers.dense(forward_fc1, s_next_conv4_flat.shape[1], activation=None)
r_i = (self.eta * 0.5) * tf.reduce_sum(tf.square(tf.subtract(forward_fc2, s_next_conv4_flat)), axis = 1)
Lf = tf.losses.mean_squared_error(forward_fc2, s_next_conv4_flat)
# Inverse Model
input_inverse = tf.concat([s_conv4_flat, s_next_conv4_flat], 1)
inverse_fc1 = tf.layers.dense(input_inverse, 256, activation=tf.nn.relu)
inverse_fc2 = tf.layers.dense(inverse_fc1, self.Num_action, activation=tf.nn.softmax)
Li = tf.losses.softmax_cross_entropy(a_t, inverse_fc2)
return s_current, s_next, a_t, r_i, Lf, Li
- 7.3 Loss
def loss_and_train(self):
# Loss function and Train
action_target = tf.placeholder(tf.float32, shape = [None, self.Num_action])
y_target = tf.placeholder(tf.float32, shape = [None])
y_prediction = tf.reduce_sum(tf.multiply(self.output, action_target), reduction_indices = 1)
Loss = self.lamb * tf.reduce_mean(tf.square(y_prediction - y_target)) + (self.beta * self.Lf) + ((1-self.beta) * self.Li)
train_step = tf.train.AdamOptimizer(learning_rate = self.learning_rate, epsilon = 1e-02).minimize(Loss)
return train_step, action_target, y_target, Loss
8、参考文献
【1】魏思齐:【李弘毅深度强化学习】7.Sparce Reward
【2】王小惟:想入歪歪,读《Curiosity-driven Exploration by Self-supervised Prediction》
请批评指正,谢谢~
编辑于 2019-11-14 <style></style>标签:self,Module,next,conv3,conv1,Curiosity,conv4,tf,Intrinsic 来源: https://www.cnblogs.com/cx2016/p/13599779.html