Pix2Pix GAN(CVPR. 2017)
作者:互联网
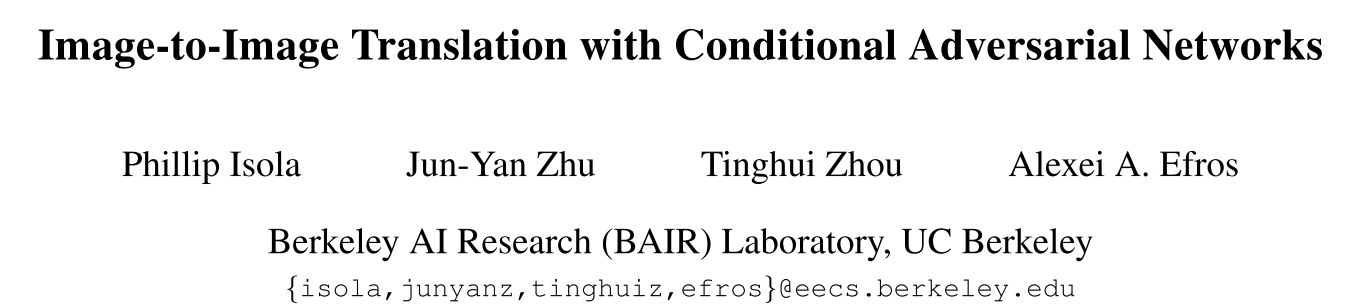
1. Motivation
Image-to-Image translation的定义
- We define automatic
image-to-image translation
as the task of translating one possible representation of a scene into another. - Our goal in this paper is to develop a common framework for all these problems.
需要认真设计loss函数,因为如果只用欧氏距离的方法,容易造成生成的图片blurry results.
- This is because Euclidean distance is minimized by averaging all plausible outputs, which causes blurring.
- Earlier papers have focused on specific applications, and it has remained unclear how effective image-conditional GANs can be as a general-purpose solution for image-to- image translation.
2. Contribution
- 本文的第一个贡献在于CGAN在多任务上可以统一,有不错的效果。
- Our primary contribution is to demonstrate that on a wide variety of problems, conditional GANs produce reasonable results.
- 本文的第二个贡献在于提出了一个简单的框架。
- Our second contribution is to present a simple framework sufficient to achieve good results, and to analyze the effects of several important architectural choices.
与之前的工作不同的是,Pix2Pix GAN在G中使用U-Net,并且在D中使用PatchGAN Classifier。
- Unlike past work, for our generator we use a “U-Net”-based architecture.
- And for our discriminator we use a convo- lutional “PatchGAN” classifier, which only penalizes struc- ture at the scale of image patches.
3. Method
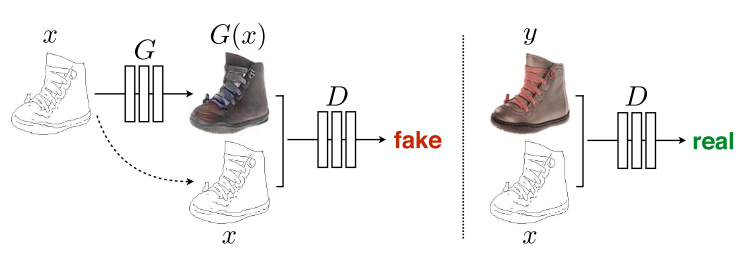
3.1 Objective
CGAN可以表示为公式1,其中x为condition:
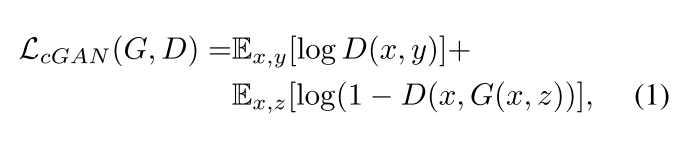
最后的目标函数表示为:

对于noise z的设定,作者采取了dropout的方式:
- Instead, for our final models, we provide noise only in the form of dropout, applied on several layers of our generator at both training and test time.
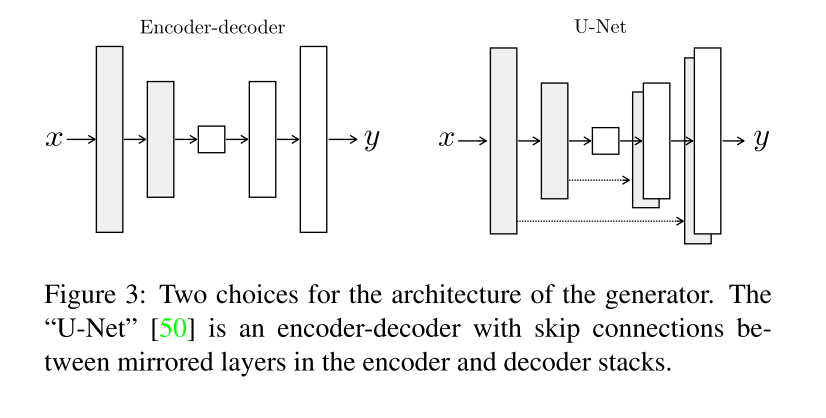
3.2 Network architecture
对于输入和输出图片来说,可以额理解为在surface appearance不同,但是具有相同的underlying structure渲染。
- In addition, for the problems we consider, the input and output differ in surface appearance, but both are renderings of the same underlying structure.
- Therefore, structure in the input is roughly aligned with structure in the output.
对于generation的制作来说,作者参考U-Net 使用了skip connection结构:
- To give the generator a means to circumvent the bottleneck for information like this, we add skip connections, fol- lowing the general shape of a “U-Net”.
3.3 Markovian discriminator (PatchGAN)
作者指出虽然L1 L2loss会使得生产的图片具有blurry模糊性质,无法捕获高频特征,但是可以精确的捕获低频的特征。这样就只需要GAN Discriminator建模高频的结构,使用L1 来建模低频。
- Although these losses fail to encourage high-frequency crispness, in many cases they nonetheless accu- rately capture the low frequencies.
那么对于制定一个建模高频的结构,在局部image patch中限制attention是有效的。因此作者制定了一个PatcchGAN,将图片分为NxN个patch,对于patches进行penalize,判断每一个patch是real还是fake。
4. Experiment
4.1 Dataset
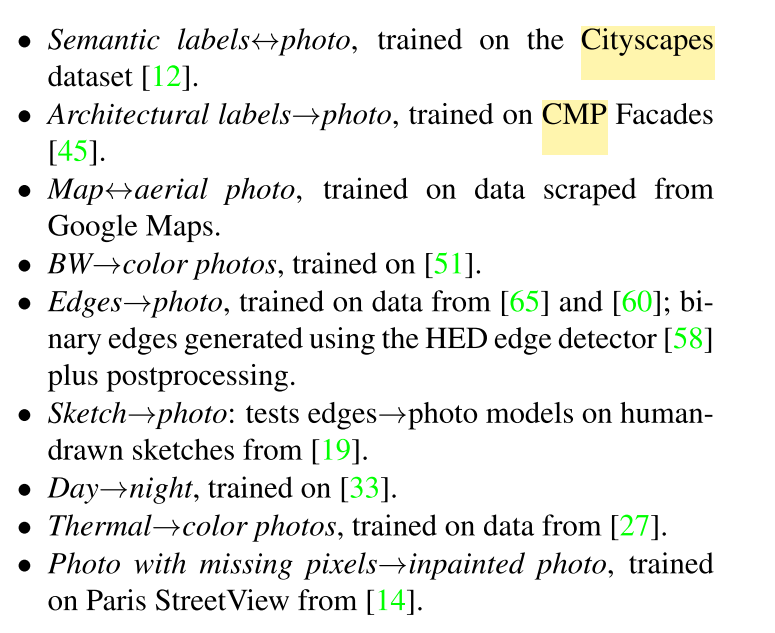
4.2 Evaluation metrics
-
We employ two tactics. First, we run “real vs. fake” perceptual studies on Amazon Mechanical Turk (AMT).
-
Second, we measure whether or not our synthesized cityscapes are realistic enough that off-the-shelf recognition system can recognize the objects in them.
-
AMT perceptual studies
-
“FCN-score”
4.3. Analysis of the generator architecture A
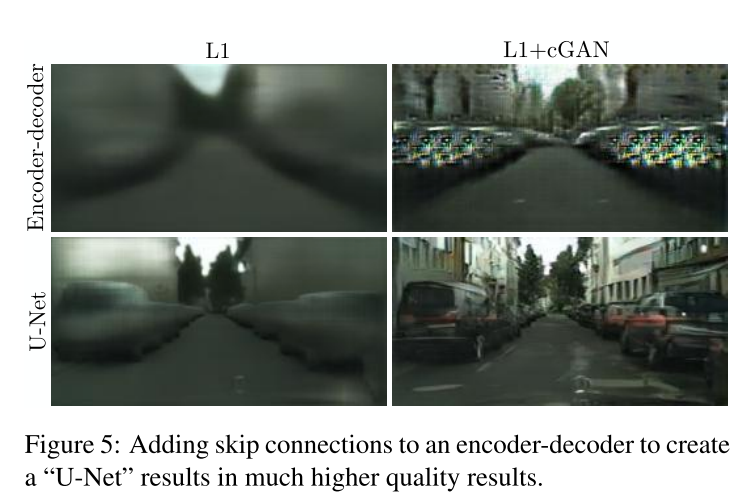
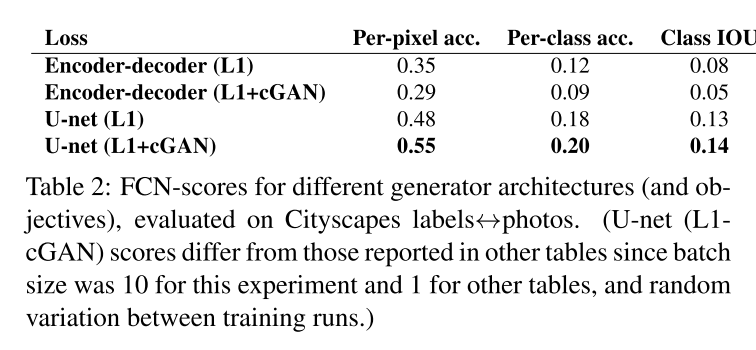
4.4 Analysis of the objective function
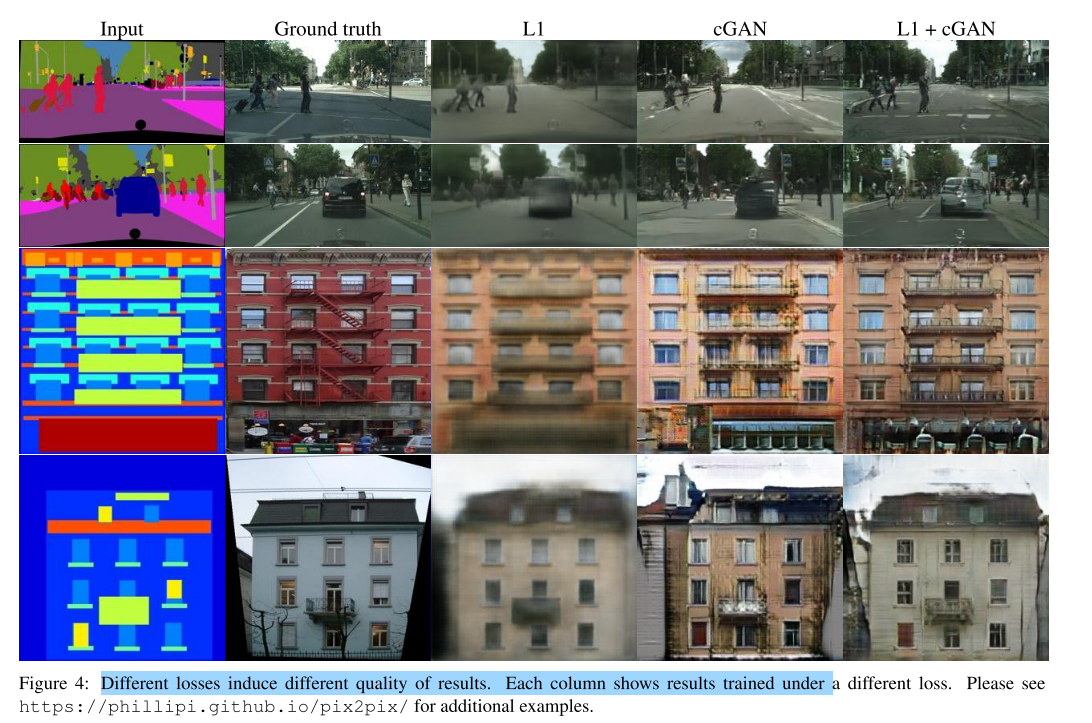
4.5 FromPixelGANs to PatchGANs to ImageGANs
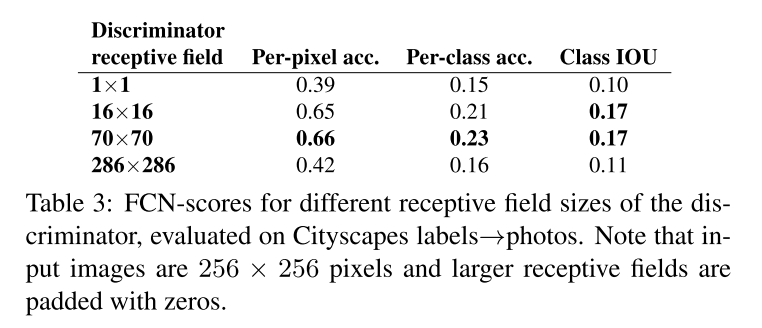
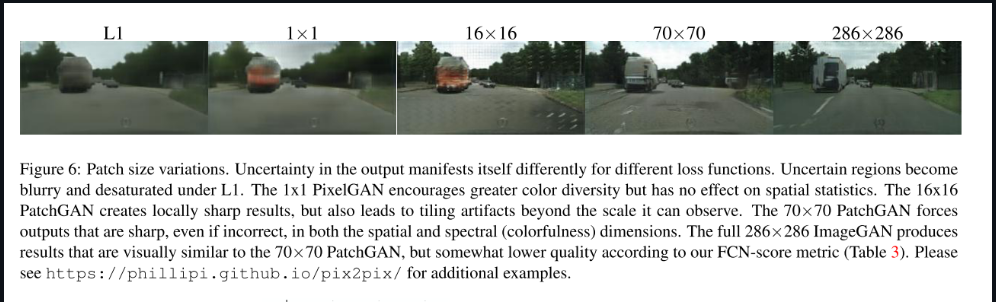
4.6. Semantic segmentation
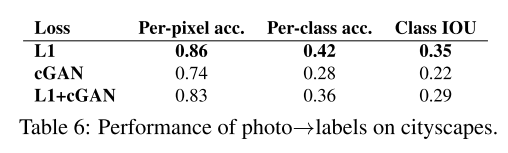
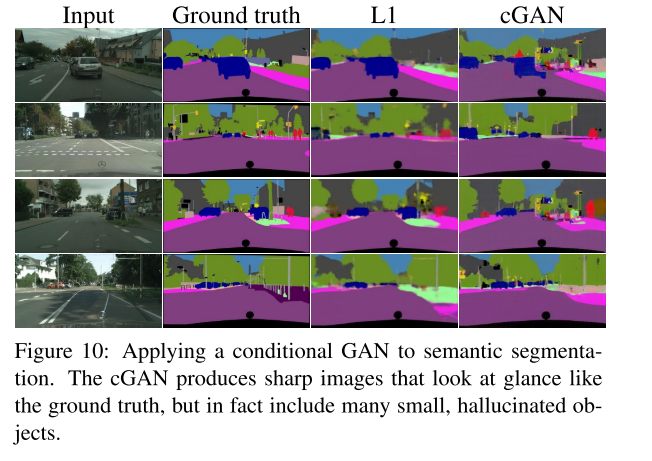
标签:generator,CVPR,image,results,Pix2Pix,our,2017,Net,structure 来源: https://blog.csdn.net/weixin_43823854/article/details/118314024